Reducing costs reactively is good. Controlling them proactively is better. And that starts with holding individuals accountable for their cloud data usage and costs.
But—and it’s a big but—if you’re going to hold individuals responsible for making better choices about their own cloud usage and costs, you have to provide them with the information that tells them what those better choices are.
And every individual incurring cloud data costs should be empowered with the information to optimize costs themselves: self-service cost optimization.
This is where most enterprise companies taking a DataFinOps approach find their biggest obstacle. “Getting engineers to take action on cost optimization” is the top challenge among FinOps organizations in general, and it’s no stretch to say that it’s even more challenging for teams running cloud data workloads.
The reason individuals—let’s say data engineers—don’t take action on cost optimization is that they don’t know what action to take. They don’t know what the “right thing to do” is. They need quite granular performance engineering details and cost calculations about how to configure their cloud data workloads and specific jobs in the most cost-effective way. Getting this information into their hands in time to avoid overspending is crucial, and can be almost impossible without AI and automation.
The vast majority of data team members have neither the time nor the expertise (background) to figure out cost optimization themselves. It might take them days or weeks (if ever) to hit upon the “right thing to do.” And the people who can figure this out are already buried under a mountain of backlog service requests.
Resolving cost issues usually relies on the same handful of scarce experts who have the know-how to resolve performance issues. In many respects, cost and performance are flip sides of the same coin—you’re looking at the same kind of granular details, stitched together into a meaningful context, only from a slightly different angle.
Automation and AI team up to deliver prescriptive cost-optimization recommendations right to every engineer’s door. Any job or workload or cluster that the AI identifies as overspending has an AI recommendation available to the engineer.
Because nobody wants a bunch of charts and graphs and metrics and billing details that still leave the heavy lifting to you. They need concrete, actionable intelligence about what to do next.
If data engineers (or any individual being held accountable for his or her cloud data usage/costs) have all this information and actionable intelligence at their disposal—automatically—then they can optimize costs themselves.
Best Practice:
Roll up all the data about individual insights and AI recommendations into an overview dashboard, something like the one below.
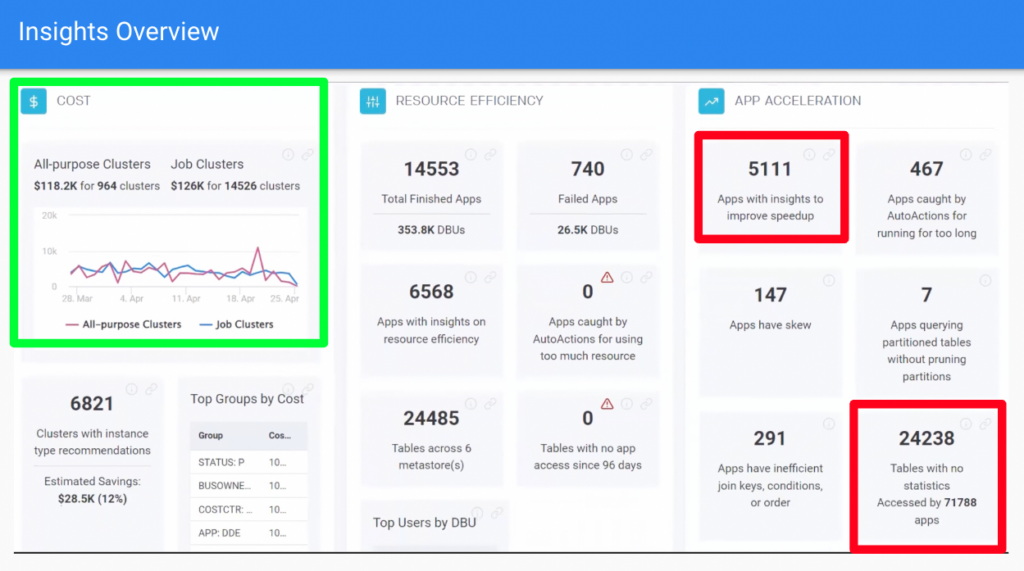
This overview lets leaders understand where cost and performance inefficiencies lie, see who is and isn’t applying cost-optimization AI recommendations (and at what rate), and measure the efficacy of the DataFinOps approach.