Usually the first questions about DataFinOps revolve around setting up or building a team to get a DataFinOps approach rolling. The practice of DataFinOps is in its early innings—DataOps is just starting to get its sea legs and along comes FinOps—so best practices are still emerging. A lot of that work is being done within the 7,000+ member FinOps Foundation, especially in the member-driven working groups (where we are now bringing all the cloud data costs considerations to the table).
You probably already have a pretty good idea of who the core key players would be. Now you’re looking to flesh out the team, expand its scope across all data teams, grow the core DataFinOps team, and otherwise operationalize DataFinOps.
Different organizations run their data workloads differently. Some places it’s “you built it, you own it,” where the data engineering development, operational responsibility, and cost management sit with the same person. Other places, these roles and responsibilities are split up: the people developing all the data applications and pipelines on one side, the people running them on another. Frequently, it’s not especially clear who owns responsibility for the cost.
No matter how your company is organized, a DataFinOps team needs some kind of representation from Finance, Data Engineering (and Operations), people from the business side like product owners, and other technical stakeholders like data architects and platform owners. Each of these groups has skin in the game for how cloud data workloads are running and how much that’s costing, and they all look at things through a slightly different lens.
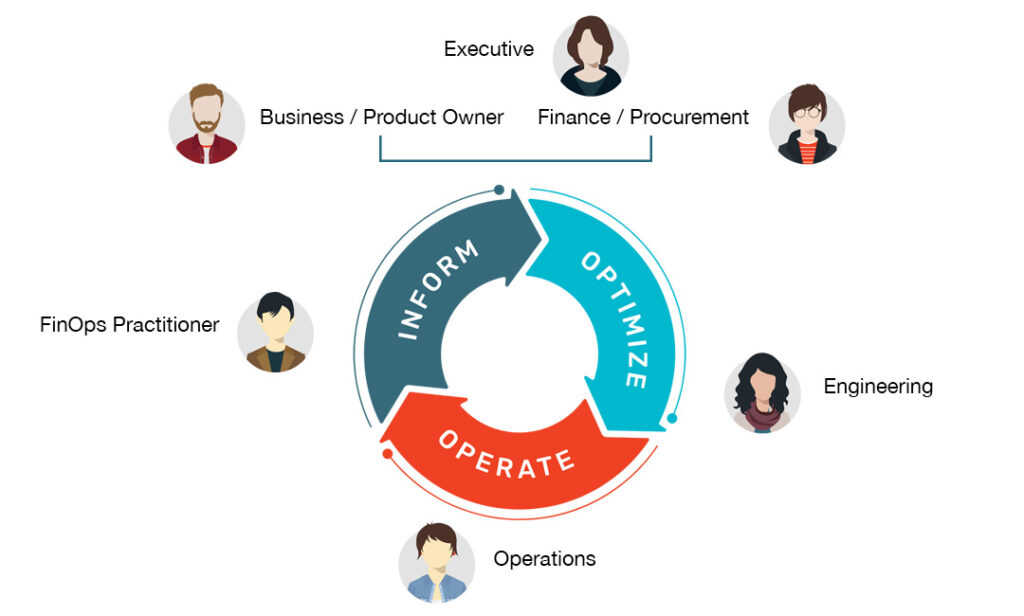
DataFinOps by Persona
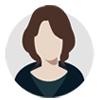
Executive
Let’s take MegaBankCorp, a fictional composite of enterprises we’ve worked with over the years that run a lot of intensive data workloads. Michael is the CDAO, and he’s the one on the hot seat at the C-suite meetings to bring their skyrocketing cloud data costs under control. His data estate is still predominantly the familiar complex sprawl of a Cloudera/Hadoop on-prem environment. But that’s getting harder and harder to manage, and they’re running out of capacity. An eight-figure capital expenditure to add more data center muscle is simply not in the cards at this time. Most of his data teams are already running pretty robust business-critical data workloads on a combination of Databricks, some Snowflake, Amazon EMR, BigQuery and Dataproc. Over the past couple of years, Michael has been migrating as many workloads over to Databricks as possible. But it’s gotten very expensive, he’s been going over budget for a while, and he’s not banking on any kind of significant budget increase
Top of mind:
Running out of budget. Intense pressure to bring cloud data costs down.
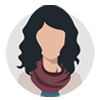
Platform Owner
One of his top data team leaders is Sammi—he’s got “data engineering” in his title, but he’s really the Databricks guy at MegaBankCorp. He’s been instrumental in migrating workloads from Hadoop to Databricks, and the bank increasingly relies on these results—for everything from fraud detection AI/ML models to preference engines to customer insights. But he sees the bills. He knows they’re paying more than they need to, but he doesn’t have the time or the people (or the people with the expertise) to find and fix all the waste and inefficiency.
Top of mind:
Databricks migration/expansion stalling out due to rising costs. Frustration at MegaBankCorp’s inability to identify and resolve cost problems at cluster level.
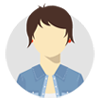
Operations
Sammi works closely with Raj, who’s headed the Ops teams for years, both for Hadoop and now the modern data stack. This is where all data workload problems come for resolution. He still spends way more time than he should have to in Hadoop, but Databricks is becoming increasingly a bigger part of his teams’ responsibility. His teams do have the kind of expertise to find and fix overspending, but nowhere near enough time. For every one of Raj’s Ops people, there are probably 10 people developing data apps and launching instances (incurring costs) in the cloud. His teams are already buried under an avalanche of trouble tickets, service requests, and other firefighting. More than anyone, he knows that it can take hours, even days, to gather and analyze all the information to figure out how to run something in the most cost-efficient manner. But there are thousands of these somethings going on every week.
Top of mind:
Already buried under deep and growing backlog, and more Databricks operational issues coming every week. Not enough people, time to get through with what’s on his plate now, let alone more.
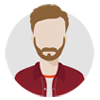
Business Owner
Jon heads up the Fraud Detection Division at MegaBankCorp, which launched a huge AI/ML initiative last year after a couple of years of fits and starts. His entire division depends on reliable data results feeding into the fraud detection model. He can’t really afford any workload failures, bottlenecks, and slowdowns; but the bank can’t afford to keep running things at any cost. He’s concerned that any cost-control initiative might compromise how quickly and reliably results get delivered.
Top of mind:
High cloud data costs impacting his division’s ability to do their jobs. Concerned that cloud data cost-cutting would put the fraud detection model at risk.
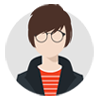
Finance
Nan is the one who pays the monthly cloud data bills. She’s watching almost every data team blow through its budget, and nobody seems to have a handle on what usage/cost will look like next month (let alone next quarter or next year). Every month the bill is a surprise, often bringing on (still) a sense of sticker shock. She struggles to forecast and set budgets. But she can see quite clearly that this type of spending cannot continue.
Top of mind:
Constant budget overruns, unpredictable usage/cost forecasting.